MCMC Example#
In this tutorial, we’ll fit the light curve of the 23 ± 4 Myr-old transiting
exoplanet star V1298 Tau with fleck
.
It is interesting to apply fleck
to V1298 Tau because it is young and
spotted, and has repeated spot signals over the duration of a few stellar
rotations. Here, we’ll use lightkurve
to download the K2 photometry,
astropy
to measure the stellar rotation period with a Lomb-Scargle,
periodogram, and finally fleck
to model the rotational modulation of the
host star in order to invert the light curve for the spot properties: latitudes,
longitudes, and radii.
First, we import all of the required packages for this tutorial:
import matplotlib.pyplot as plt
import numpy as np
from astropy.coordinates import SkyCoord
from astropy.timeseries import LombScargle
import astropy.units as u
import healpy as hp
from lightkurve import search_lightcurve
from emcee import EnsembleSampler
from multiprocessing import Pool
from corner import corner
from fleck import Star
Note
These examples require a few extra dependencies, get them with:
pip install fleck[docs]
Next we resolve the target coordinates using astropy, and download its light
curve using lightkurve
’s handy method:
coord = SkyCoord.from_name('V1298 Tau')
slcf = search_lightcurve(coord, mission='K2')
lc = slcf.download_all()
pdcsap = lc.PDCSAP_FLUX.stitch()
time = pdcsap.time
flux = pdcsap.flux
notnanflux = ~np.isnan(flux)
flux = flux[notnanflux & (time > 2230) & (time < 2239)]
time = time[notnanflux & (time > 2230) & (time < 2239)]
flux /= np.mean(flux)
Next, we want to determine the stellar rotation period, which will remain fixed
in our fleck
analysis. We do this with a Lomb-Scargle periodogram:
periods = np.linspace(1, 5, 1000) * u.day
freqs = 1 / periods
powers = LombScargle(time * u.day, flux).power(freqs)
best_period = periods[powers.argmax()]
Now that we know that the best period is roughly 2.87 days, we can generate
fleck
light curves that reproduce the spot modulation:
u_ld = [0.46, 0.11]
contrast = 0.7
phases = (time % best_period.value) / best_period.value
s = Star(contrast, u_ld, n_phases=len(time), rotation_period=best_period.value)
init_lons = np.array([0, 320, 100])
init_lats = [0, 20, 0]
init_rads = [0.01, 0.2, 0.3]
yerr = 0.002
init_p = np.concatenate([init_lons, init_lats, init_rads])
With our initial parameters set, we now define the functions that emcee
uses
to do Markov Chain Monte Carlo:
def log_likelihood(p):
lons = p[0:3]
lats = p[3:6]
rads = p[6:9]
lc = s.light_curve(lons[:, None] * u.deg, lats[:, None] * u.deg, rads[:, None],
inc_stellar=90*u.deg, times=time, time_ref=0)[:, 0]
return - 0.5 * np.sum((lc/np.mean(lc) - flux)**2 / yerr**2)
def log_prior(p):
lons = p[0:3]
lats = p[3:6]
rads = p[6:9]
if (np.all(rads < 1.) and np.all(rads > 0) and np.all(lats > -60) and
np.all(lats < 60) and np.all(lons > 0) and np.all(lons < 360)):
return 0
return -np.inf
def log_probability(p):
lp = log_prior(p)
if not np.isfinite(lp):
return -np.inf
return lp + log_likelihood(p)
We’ll run the chains for a short period of time to see the results faster, but to ensure convergence, you should run for 10-100x longer than this example:
ndim = len(init_p)
nwalkers = 5 * ndim
nsteps = 5000
pos = []
while len(pos) < nwalkers:
trial = init_p + 0.01 * np.random.randn(ndim)
lp = log_prior(trial)
if np.isfinite(lp):
pos.append(trial)
with Pool() as pool:
sampler = EnsembleSampler(nwalkers, ndim, log_probability, pool=pool)
sampler.run_mcmc(pos, nsteps, progress=True);
samples_burned_in = sampler.flatchain[len(sampler.flatchain)//2:, :]
Now let’s plot the corner plot with the posterior distributions and their
correlations using the corner
package:
fig, ax = plt.subplots(9, 9, figsize=(12, 12))
labels = "lon0 lon1 lon2 lat0 lat1 lat2 rad0 rad1 rad2".split()
corner(samples_burned_in, smooth=True, labels=labels,
fig=fig);
plt.show()
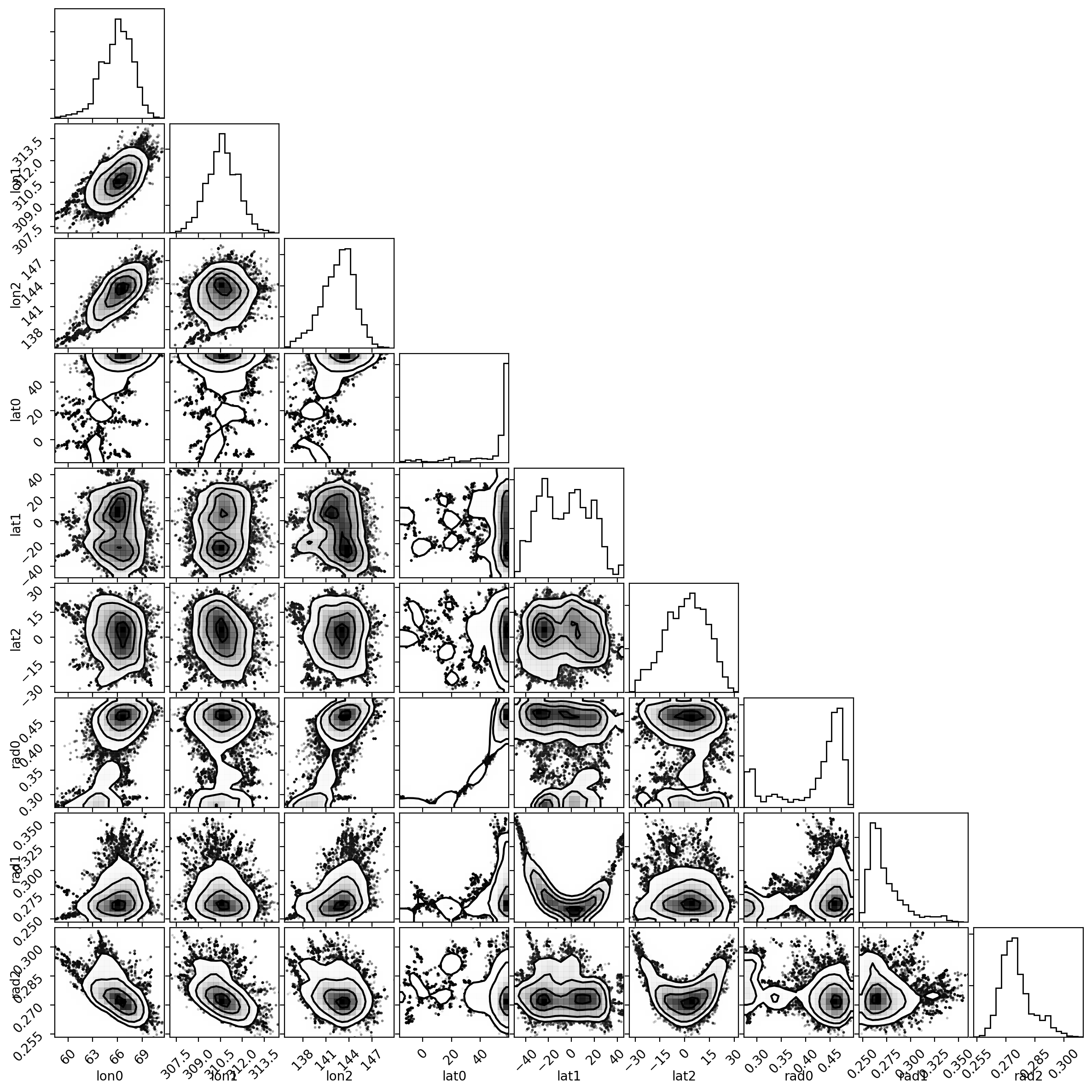
Finally, let’s plot several draws from the posterior distributions for near the
maximum-likelihood light curve model, the total spot coverage posterior
distribution, and the spot map using healpy
:
fig, ax = plt.subplots(1, 3, figsize=(8, 1.5))
for i in np.random.randint(0, len(samples_burned_in), size=50):
trial = samples_burned_in[i, :]
lons = trial[0:3]
lats = trial[3:6]
rads = trial[6:9]
lc = s.light_curve(lons[:, None] * u.deg, lats[:, None] * u.deg, rads[:, None],
inc_stellar=90*u.deg, times=time, time_ref=0)[:, 0]
ax[0].plot(time, lc/lc.mean(), color='DodgerBlue', alpha=0.05)
f_S = np.sum(samples_burned_in[:, -3:]**2 / 4, axis=1)
ax[1].hist(f_S, bins=25, histtype='step', lw=2, color='k', range=[0, 0.12], density=True)
ax[0].set(xlabel='BJD - 2454833', ylabel='Flux', xticks=[2230, 2233, 2236, 2239])
ax[1].set_xlabel('$f_S$')
ax[0].plot(time, flux, '.', ms=2, color='k', zorder=10)
NSIDE = 2**10
NPIX = hp.nside2npix(NSIDE)
m = np.zeros(NPIX)
np.random.seed(0)
random_index = np.random.randint(samples_burned_in.shape[0]//2,
samples_burned_in.shape[0])
random_sample = samples_burned_in[random_index].reshape((3, 3)).T
for lon, lat, rad in random_sample:
t = np.radians(lat + 90)
p = np.radians(lon)
spot_vec = hp.ang2vec(t, p)
ipix_spots = hp.query_disc(nside=NSIDE, vec=spot_vec, radius=rad)
m[ipix_spots] = 0.7
cmap = plt.cm.Greys
cmap.set_under('w')
plt.axes(ax[2])
hp.mollview(m, cbar=False, title="", cmap=cmap, hold=True,
max=1.0, notext=True, flip='geo')
hp.graticule(color='silver')
fig.suptitle('V1298 Tau')
for axis in ax:
for sp in ['right', 'top']:
axis.spines[sp].set_visible(False)
plt.show()
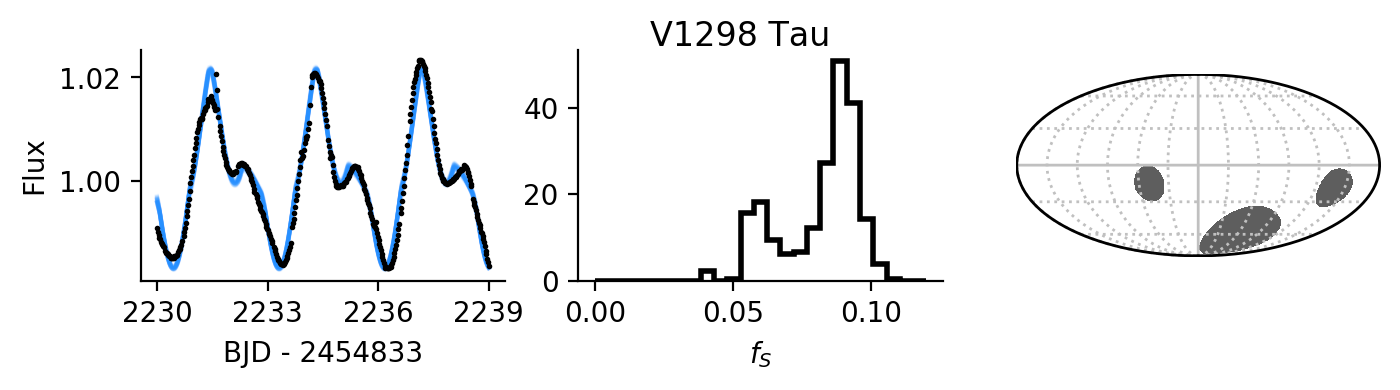
Finally, we can print the spot coverage:
lo, mid, hi = np.percentile(f_S, [16, 50, 84])
print(f"$f_S = {{{mid:g}}}^{{+{hi-mid:g}}}_{{-{mid-lo:g}}}$")
which returns \(f_S = {0.087}^{+0.006}_{-0.025}\).